Building Trust in Artificial Intelligence
As Artificial Intelligence (AI) continues to influence every aspect of our lives, from healthcare to finance, one critical question arises: Can we trust these intelligent systems? While AI excels at making predictions and decisions, the “black box” nature of many models makes understanding their reasoning a challenge. This lack of transparency can lead to mistrust, unintended biases, and even harmful consequences.
Explainable AI (XAI) and transparent algorithms aim to address this challenge by making AI systems more interpretable, trustworthy, and accountable. In this blog, we’ll explore what XAI is, its significance, applications, and the challenges it faces.(Building Trust in Artificial Intelligence)
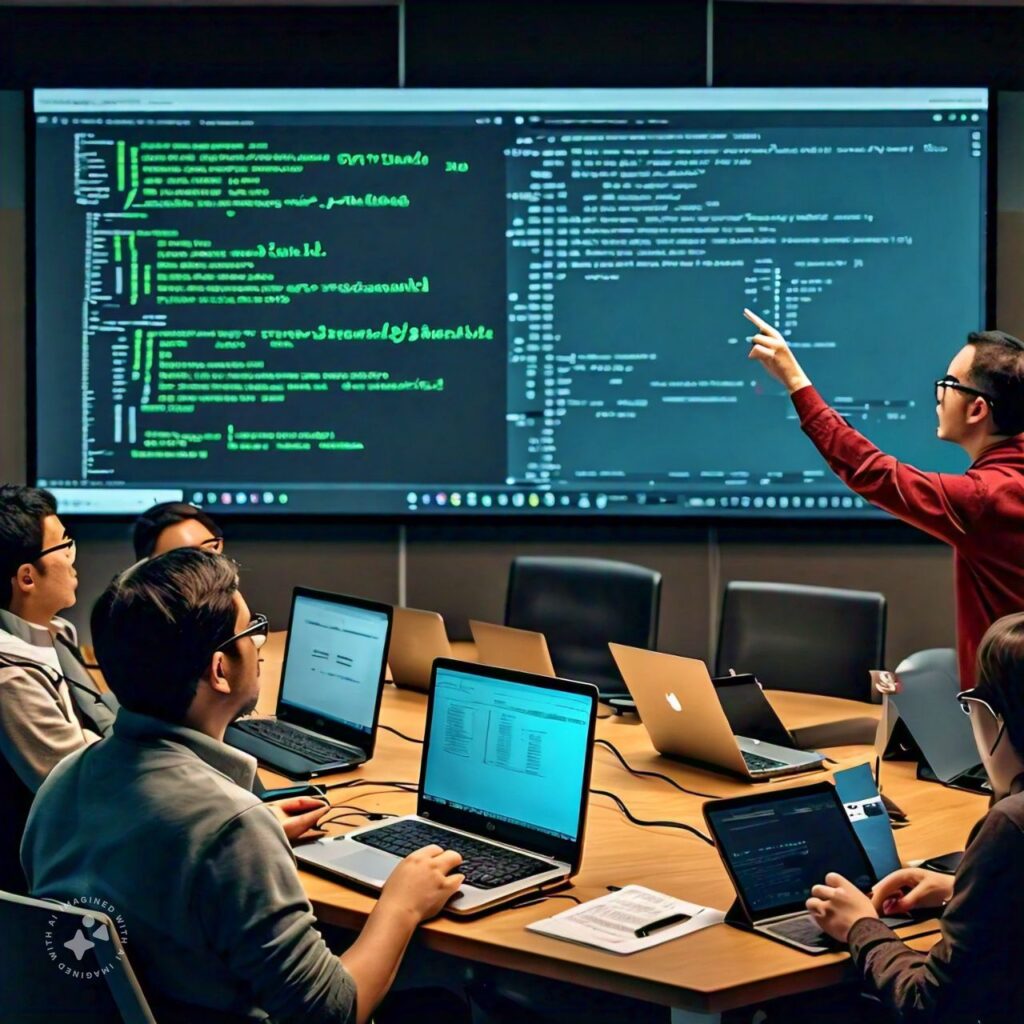
What is Explainable AI (XAI)?
Explainable AI refers to methods and techniques that enable humans to understand and trust the outputs of AI models. XAI ensures that AI systems provide not just results but also clear explanations for how those results were reached.
Key Features of XAI:
- Transparency: Clear insights into how the AI system works.
- Interpretability: Simplifying complex models into human-understandable explanations.
- Accountability: Ensuring ethical and fair decision-making.
For example, in a credit scoring system, XAI can explain why a loan was approved or denied, detailing which factors contributed most to the decision.(Building Trust in Artificial Intelligence)
Why is Explainable AI Important?
1. Building Trust
Transparent algorithms help users understand and trust AI decisions, especially in high-stakes scenarios like healthcare or law enforcement.
2. Ensuring Fairness
XAI identifies and mitigates biases in AI systems, ensuring equitable outcomes across diverse populations.
3. Enhancing Compliance
Regulatory frameworks, such as GDPR, demand accountability and transparency in automated decision-making. XAI helps organizations comply with these regulations.
4. Improving AI Models
By understanding an AI system’s behavior, developers can identify weaknesses, optimize performance, and enhance reliability.(Building Trust in Artificial Intelligence)
How Transparent Algorithms Work
Transparent algorithms are designed to be inherently interpretable. Unlike black-box models such as deep neural networks, transparent models provide clear and concise outputs. Techniques for achieving transparency include:
1. Feature Importance Analysis
Identifying which inputs (features) influence a model’s decision most.
- Example: In a predictive healthcare model, age and smoking status might be identified as key factors for heart disease risk.
2. Model-Agnostic Methods
Techniques like LIME (Local Interpretable Model-agnostic Explanations) and SHAP (SHapley Additive exPlanations) provide explanations for predictions, regardless of the underlying model.
3. Rule-Based Models
Decision trees and rule-based systems inherently provide a step-by-step explanation of how decisions are made.
4. Visualization Tools
Graphical representations of decision-making processes, such as heatmaps or attention maps, help users understand AI behavior.(Building Trust in Artificial Intelligence)
Applications of Explainable AI
1. Healthcare
In medical diagnosis, XAI helps doctors understand why an AI system predicts certain conditions, ensuring that decisions are accurate and justifiable.
- Example: AI detecting cancer in X-rays can highlight the regions contributing to its prediction.
2. Finance
XAI is critical in financial institutions to justify credit approvals, detect fraudulent transactions, and comply with regulatory requirements.
- Example: Banks use XAI to explain why a loan applicant was denied, based on income, credit history, and debt-to-income ratio.
3. Autonomous Vehicles
XAI ensures that autonomous systems can explain their decisions, such as why a car slowed down or took a particular route, enhancing safety and accountability.
4. Legal Systems
AI models used in sentencing or bail decisions must explain their reasoning to ensure fairness and avoid biases.(Building Trust in Artificial Intelligence)
Benefits of Explainable AI
- Enhanced Trust: Users feel confident in systems that justify their actions.
- Improved Adoption: Businesses are more likely to deploy AI if its decisions are transparent and interpretable.
- Bias Reduction: XAI exposes and addresses potential biases in AI models.
- Regulatory Compliance: Ensures adherence to legal requirements like GDPR or CCPA.(Building Trust in Artificial Intelligence)
Challenges in Explainable AI
- Complexity of Models: Highly accurate models like deep learning are often difficult to interpret without compromising performance.
- Trade-Off Between Accuracy and Interpretability: Simplifying models for transparency may reduce their predictive power.
- Standardization: A lack of universal standards for explainability makes it challenging to implement XAI consistently.
- Human Bias in Interpretation: Even with explanations, users might misinterpret or distrust the AI’s reasoning.(Building Trust in Artificial Intelligence)
The Future of XAI and Transparent Algorithms
As AI becomes more pervasive, the demand for explainability will grow. Future advancements in XAI may include:
- Hybrid Models: Combining interpretable models with high-performing black-box systems for the best of both worlds.
- Interactive Explanations: AI systems that provide dynamic, user-specific explanations.
- Ethical AI Frameworks: Developing global standards to ensure fairness, transparency, and accountability.
- Human-AI Collaboration: Enhancing explainability to foster better collaboration between humans and machines.(Building Trust in Artificial Intelligence)
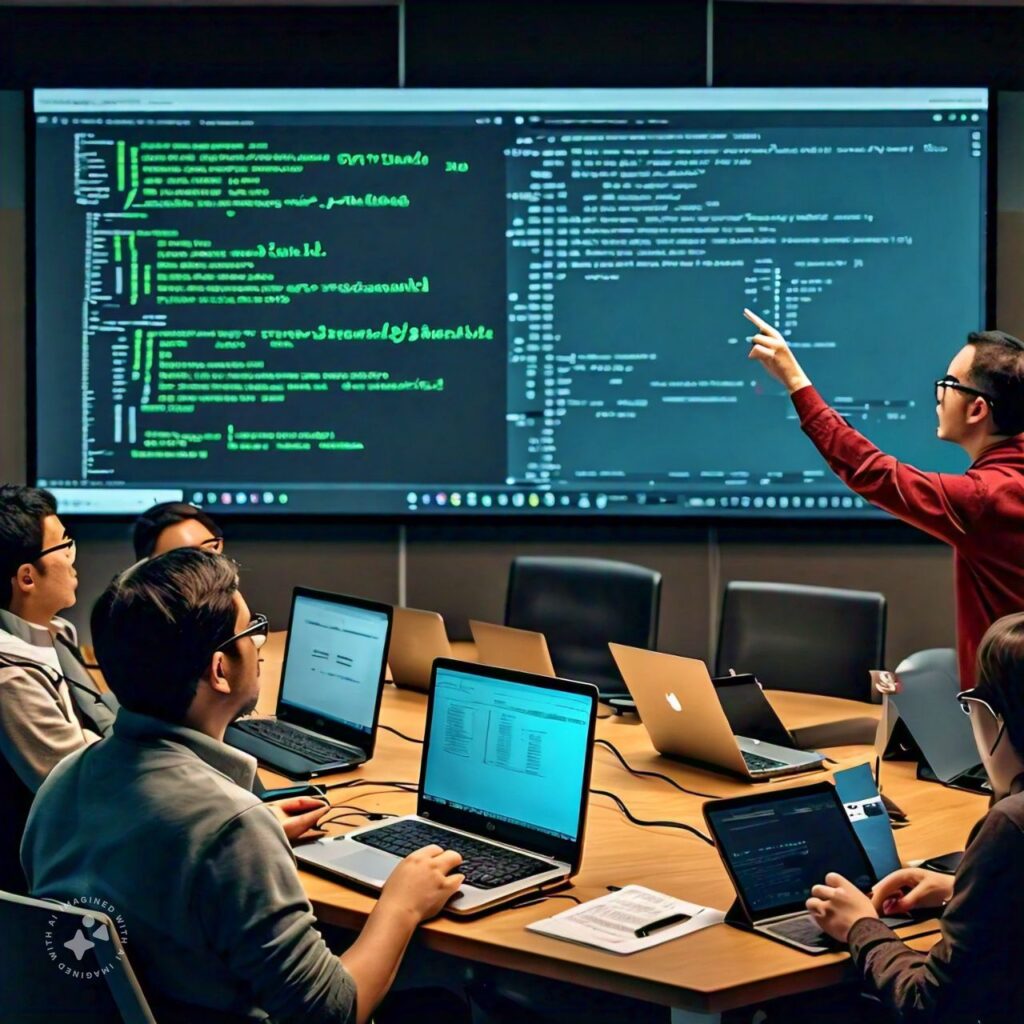
Conclusion
Explainable AI and transparent algorithms are crucial for the responsible deployment of AI technologies. They bridge the gap between complex machine learning systems and human understanding, fostering trust, fairness, and accountability.
As AI continues to evolve, organizations must prioritize explainability to ensure their systems are not only effective but also ethical and transparent. By adopting XAI, businesses can enhance their reputation, comply with regulations, and build systems that truly serve humanity.(Building Trust in Artificial Intelligence)
Social Media handles (Facebook, Linkedin, Twitter
Go to our Website for News and Articles: https://informtoyou.com/