Bioinformatics Algorithms How computers
In the age of big data, the field of bioinformatics has emerged as a crucial intersection of biology and computer science. Bioinformatics algorithms are the computational tools that enable scientists to analyze and interpret vast amounts of biological data, such as DNA sequences, protein structures, and complex biomolecular interactions. These algorithms integrate mathematics, statistics, and computer science to address intricate biological problems, transforming raw data into meaningful insights. (Bioinformatics Algorithms How computers)

The Role of Bioinformatics in Modern Biology
Bioinformatics plays a pivotal role in modern biology by providing the tools necessary to manage and analyze the enormous datasets generated by high-throughput technologies. For instance, the sequencing of the human genome, which once took years and the collaboration of numerous international research organizations, can now be completed in a matter of days thanks to advancements in sequencing technologies and bioinformatics1. (Bioinformatics Algorithms How computers)
Key Bioinformatics Algorithms
- Sequence Alignment Algorithms: These algorithms are fundamental in bioinformatics, used to identify regions of similarity between DNA, RNA, or protein sequences. The Needleman-Wunsch algorithm for global alignment and the Smith-Waterman algorithm for local alignment are classic examples. These algorithms help in identifying homologous sequences, predicting functions of unknown genes, and understanding evolutionary relationships.
- Hidden Markov Models (HMMs): HMMs are used in various bioinformatics applications, including gene prediction and protein structure prediction. They model biological sequences as probabilistic processes, allowing for the identification of genes and regulatory elements within a genome.
- Clustering Algorithms: These algorithms group similar data points together and are widely used in gene expression analysis. Techniques such as k-means clustering and hierarchical clustering help in identifying patterns in gene expression data, which can lead to the discovery of gene functions and regulatory mechanisms.
- Phylogenetic Tree Construction: Algorithms like Neighbor-Joining and Maximum Likelihood are used to construct phylogenetic trees, which represent the evolutionary relationships between different species or genes. These trees are essential for understanding the evolutionary history of organisms and the genetic basis of diseases.
- Machine Learning Algorithms: With the advent of big data, machine learning has become increasingly important in bioinformatics. Algorithms such as support vector machines (SVMs), neural networks, and random forests are used for tasks like protein structure prediction, disease classification, and biomarker discovery. (Bioinformatics Algorithms How computers)
Applications of Bioinformatics Algorithms
- Genomics: Bioinformatics algorithms are essential for analyzing genomic data. They help in identifying genes, predicting their functions, and understanding genetic variations. For example, genome-wide association studies (GWAS) use statistical algorithms to identify genetic variants associated with diseases.
- Proteomics: In proteomics, bioinformatics algorithms are used to analyze protein sequences and structures. Techniques like mass spectrometry generate large datasets that require sophisticated algorithms for protein identification and quantification.
- Systems Biology: Bioinformatics algorithms are used to model and analyze complex biological systems. By integrating data from genomics, proteomics, and other fields, these algorithms help in understanding the interactions within biological networks and predicting the behavior of biological systems. (Bioinformatics Algorithms How computers)
- Drug Discovery: Bioinformatics plays a crucial role in drug discovery by identifying potential drug targets and predicting the effects of drug candidates. Algorithms are used to screen large libraries of compounds, model drug-receptor interactions, and optimize drug design.
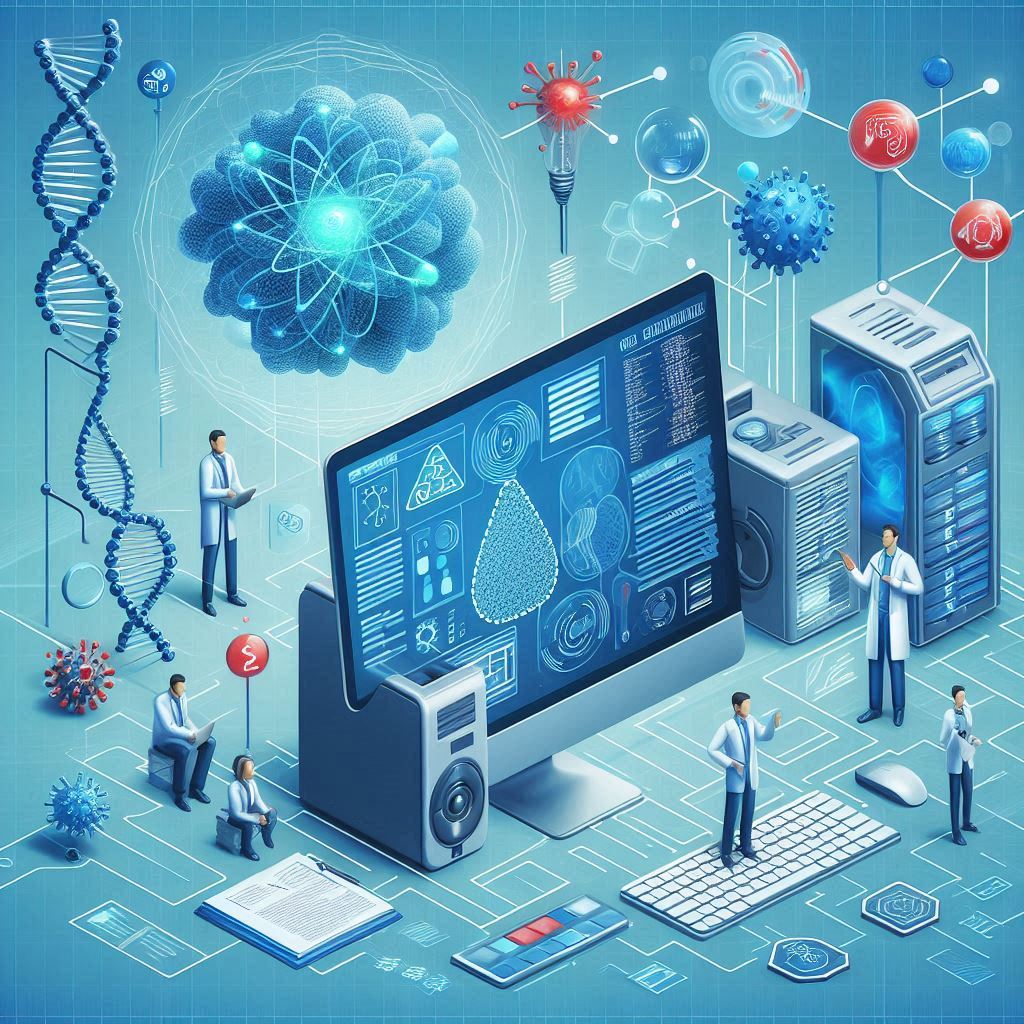
Challenges and Future Directions
Despite the significant advancements, bioinformatics faces several challenges. The sheer volume and complexity of biological data require efficient algorithms and powerful computational resources. Additionally, the integration of heterogeneous data from different sources remains a major challenge. (Bioinformatics Algorithms How computers)
Looking ahead, the future of bioinformatics lies in the development of more sophisticated algorithms and the integration of artificial intelligence (AI) and machine learning. These technologies have the potential to revolutionize bioinformatics by providing more accurate predictions and uncovering new insights from biological data. (Bioinformatics Algorithms How computers)
Conclusion
Bioinformatics algorithms are indispensable tools in modern biology, enabling the analysis and interpretation of vast amounts of biological data. From sequence alignment to machine learning, these algorithms have transformed our understanding of biology and opened new avenues for research and discovery. As technology continues to advance, bioinformatics will undoubtedly play an even more critical role in the life sciences, driving innovations in genomics, proteomics, systems biology, and beyond. (Bioinformatics Algorithms How computers)
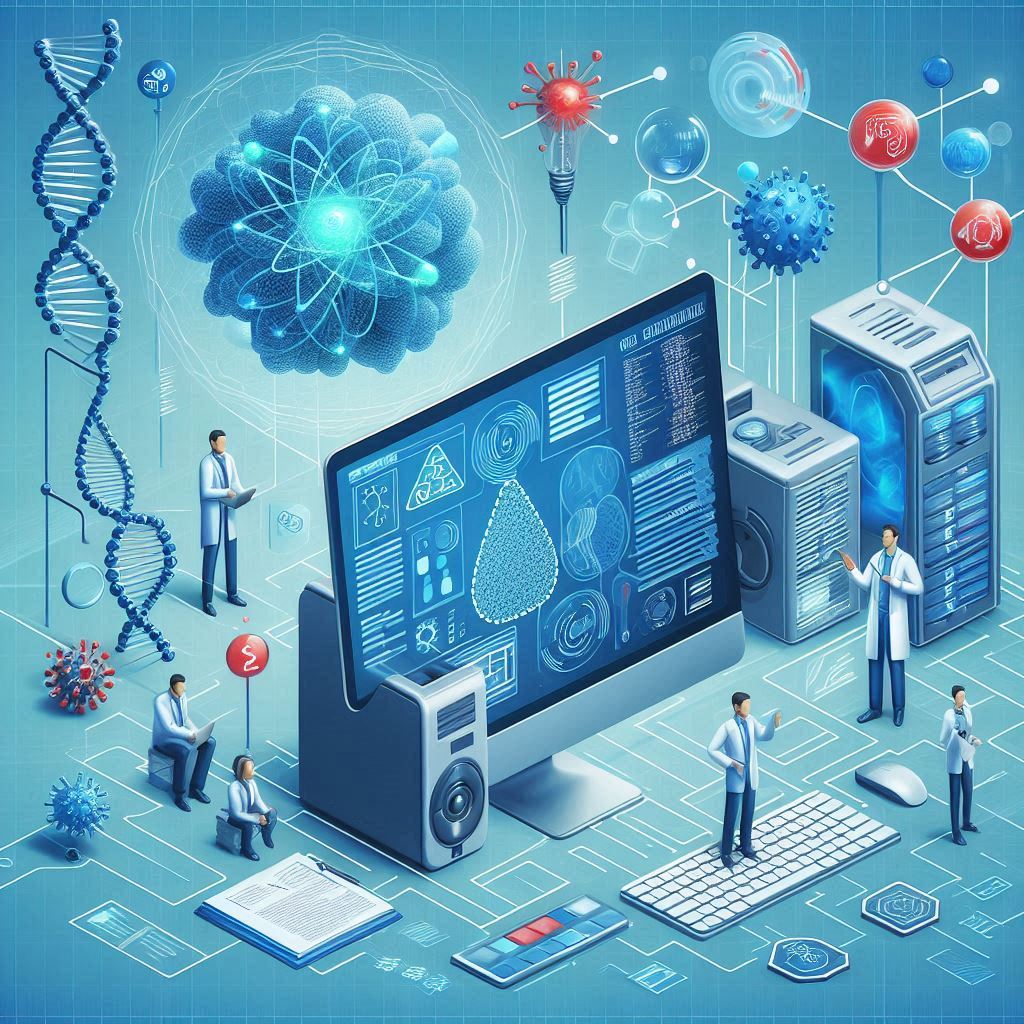
Social Media handles (Facebook, Linkedin, Twitter
Go to our Website for News and Articles: https://informtoyou.com/