AI in Drug Discovery and Pharmaceutical Research
The pharmaceutical industry is at the forefront of innovation, constantly seeking faster, more efficient ways to develop life-saving treatments. However, the traditional drug discovery process is lengthy, costly, and often fraught with failures. Enter Artificial Intelligence (AI)—a revolutionary tool transforming how new drugs are discovered, tested, and brought to market.
In this blog, we’ll delve into the transformative role of AI in drug discovery and pharmaceutical research, highlighting its potential to revolutionize healthcare.(AI in Drug Discovery and Pharmaceutical Research)
Challenges in Traditional Drug Discovery
Drug discovery is a complex, multi-step process that includes:
- Target Identification: Identifying biological molecules associated with a disease.
- Lead Compound Discovery: Finding molecules that interact with the target to produce the desired therapeutic effect.
- Preclinical Testing: Testing on cell cultures and animal models.
- Clinical Trials: Assessing safety and efficacy in humans.
This process can take 10–15 years and costs billions of dollars. Moreover, a high percentage of drugs fail during clinical trials, adding to the financial and time burden.(AI in Drug Discovery and Pharmaceutical Research)
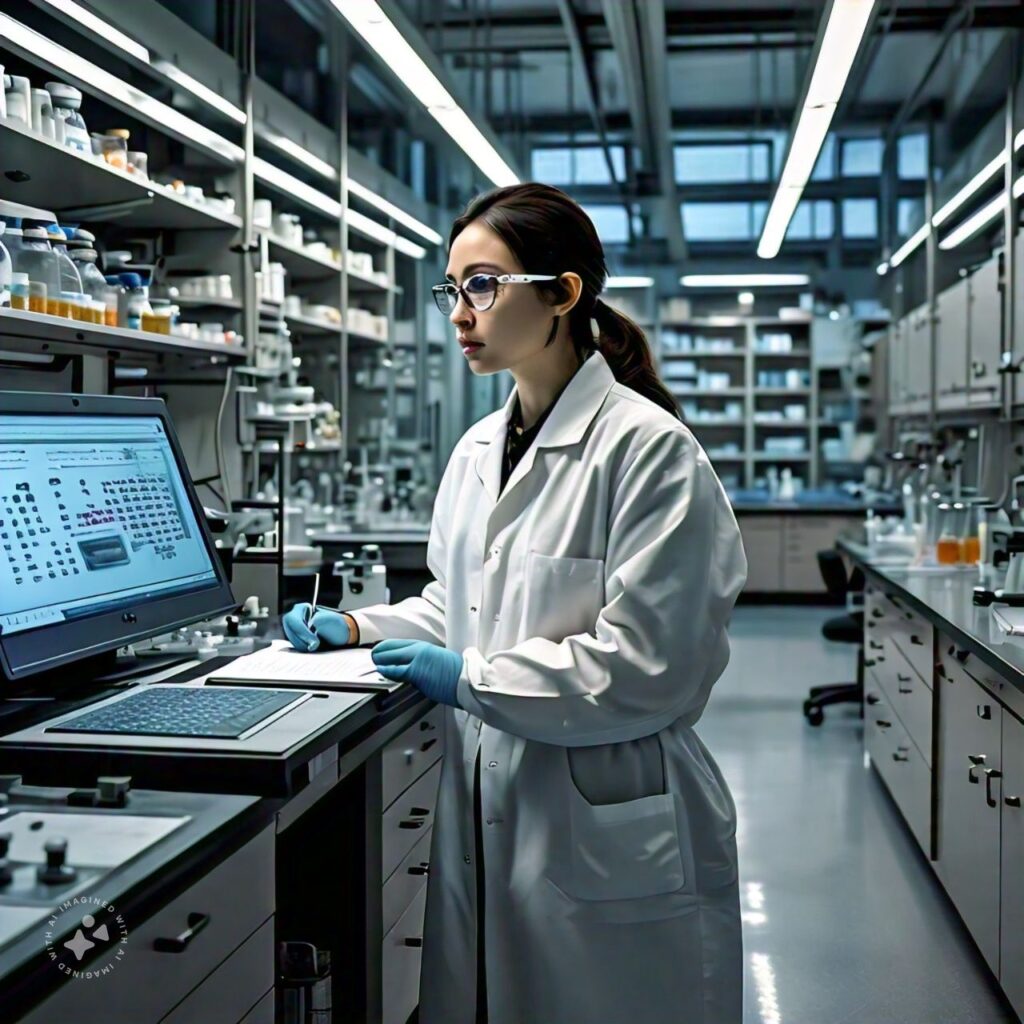
AI: The Game-Changer in Drug Discovery
AI is revolutionizing the pharmaceutical industry by addressing these challenges. With its ability to process massive datasets, predict outcomes, and identify patterns, AI is transforming drug discovery in the following ways:
1. Accelerating Target Identification
AI systems analyze genomic, proteomic, and clinical data to identify potential drug targets with high precision.
- Example: AI algorithms identify disease-associated genes or proteins, prioritizing the most promising targets for drug development.(AI in Drug Discovery and Pharmaceutical Research)
2. Enhancing Lead Compound Discovery
AI models predict which chemical compounds are likely to bind to a target effectively. By simulating these interactions virtually, researchers can screen millions of compounds in a fraction of the time required by traditional methods.
- Example: Deep learning models predict drug-protein binding affinities, reducing the need for physical testing.(AI in Drug Discovery and Pharmaceutical Research)
3. Predicting Drug Efficacy and Toxicity
AI algorithms analyze historical data to predict the safety and efficacy of drug candidates, reducing the likelihood of failures in clinical trials.
- Example: Machine learning models flag potential side effects early in the development process.
4. Streamlining Clinical Trials
AI helps design smarter clinical trials by:
- Identifying suitable participants based on genetic and health data.
- Predicting outcomes to optimize trial protocols.
- Monitoring patient responses in real time.
5. Drug Repurposing
AI identifies new uses for existing drugs by analyzing large datasets of biological and chemical information.
- Example: During the COVID-19 pandemic, AI models were used to identify existing drugs that could be repurposed to combat the virus.(AI in Drug Discovery and Pharmaceutical Research)
Applications of AI in Pharmaceutical Research
1. Personalized Medicine
AI enables the creation of personalized treatment plans by analyzing patient-specific data, such as genetic profiles and medical histories.
- Example: AI-driven platforms suggest tailored cancer treatments based on individual tumor profiles.(AI in Drug Discovery and Pharmaceutical Research)
2. Virtual Screening
AI-powered virtual screening platforms predict how thousands of compounds will interact with a biological target, drastically speeding up the discovery process.
3. Molecular Design
Generative AI models create novel molecules with desired properties, paving the way for entirely new classes of drugs.
- Example: Generative adversarial networks (GANs) design compounds with optimal pharmacological characteristics.(AI in Drug Discovery and Pharmaceutical Research)
4. Real-Time Data Analysis
AI analyzes real-world data, such as electronic health records and wearable device data, to identify patterns that inform drug development and disease management.
Benefits of AI in Drug Discovery
- Speed: AI accelerates every step of the drug development pipeline, significantly reducing the time to market.
- Cost-Effectiveness: By identifying failures early, AI reduces the financial risks associated with drug development.
- Accuracy: AI’s predictive power enhances the precision of drug discovery, minimizing trial-and-error.
- Scalability: AI can analyze vast datasets, enabling researchers to tackle complex diseases with large-scale studies.(AI in Drug Discovery and Pharmaceutical Research)
Challenges of Using AI in Drug Discovery
While AI holds immense promise, its integration into pharmaceutical research is not without challenges:
- Data Quality: AI models require high-quality, standardized data, which is often difficult to obtain.
- Regulatory Hurdles: Ensuring compliance with stringent regulatory requirements can slow down AI implementation.
- Interpretability: Many AI models operate as “black boxes,” making it difficult to explain their decisions.
- Skill Gap: The pharmaceutical industry faces a shortage of AI-trained professionals.
Success Stories: AI in Action
- DeepMind’s AlphaFold: Solved the protein-folding problem, providing insights into protein structures that aid drug design.
- Insilico Medicine: Used AI to identify a new drug candidate for idiopathic pulmonary fibrosis in just 18 months.
- Atomwise: Its AI platform predicted molecules for Ebola treatment, drastically reducing research timelines.(AI in Drug Discovery and Pharmaceutical Research)
The Future of AI in Pharmaceuticals
The integration of AI into pharmaceutical research is still in its early stages, but its potential is vast:
- Predictive Medicine: AI will play a key role in predicting disease outbreaks and treatment responses.
- Advanced Molecular Design: AI-driven tools will create increasingly complex and effective drugs.
- Global Collaboration: AI platforms will facilitate collaboration across organizations and countries, accelerating innovation.(AI in Drug Discovery and Pharmaceutical Research)
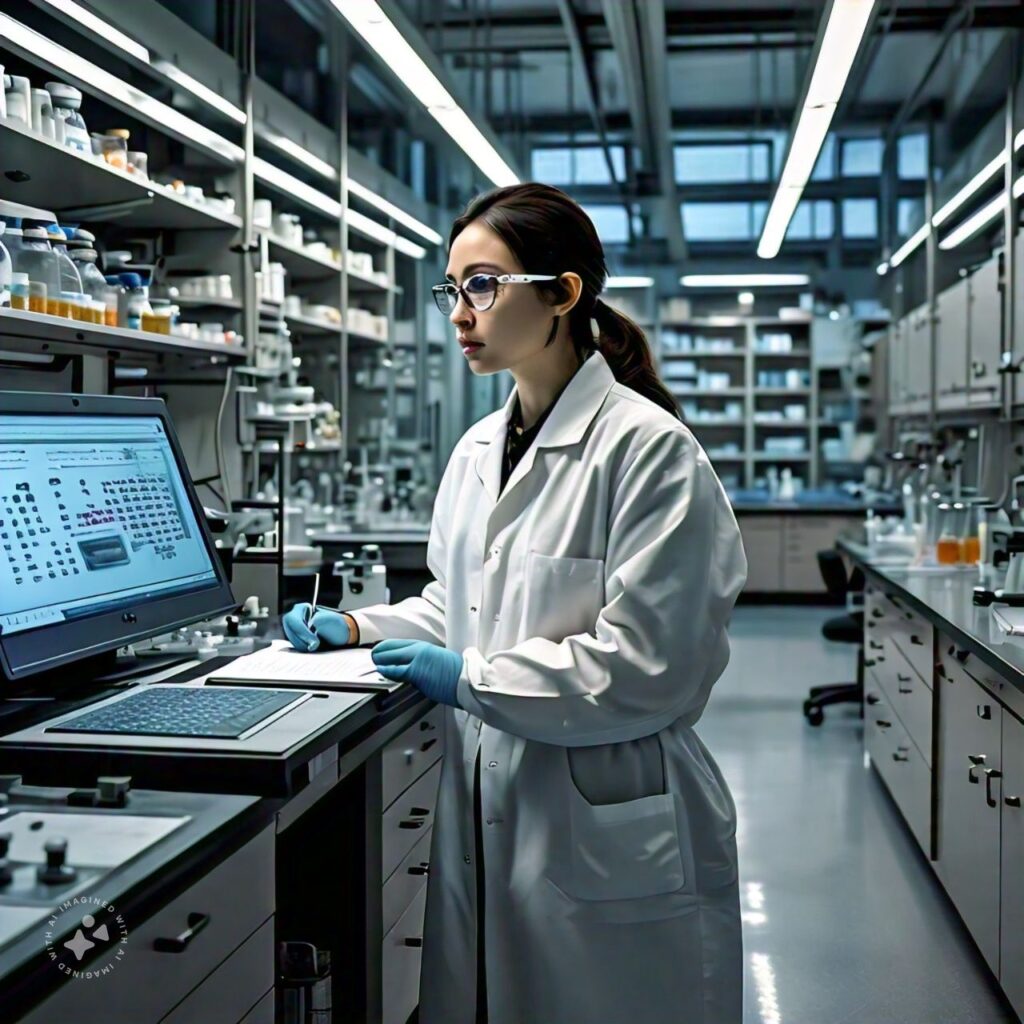
Conclusion
AI is reshaping the landscape of drug discovery and pharmaceutical research, offering unprecedented speed, accuracy, and cost-efficiency. While challenges remain, the success stories and rapid advancements in AI-driven technologies underscore its transformative potential.
As AI continues to mature, its role in combating complex diseases and improving global healthcare outcomes will only grow. For pharmaceutical companies, embracing AI is no longer optional—it’s a necessity for staying ahead in the race to develop life-saving treatments.(AI in Drug Discovery and Pharmaceutical Research)
Social Media handles (Facebook, Linkedin, Twitter
Go to our Website for News and Articles: https://informtoyou.com/